Machine learning techniques to support on-farm yield adaptability
The relationship between biophysical (environmental conditions) and crop management variables can explain 25% yield variability. This was revealed by a study using interpretable machine learning methods using large-scale farmers’ field data.
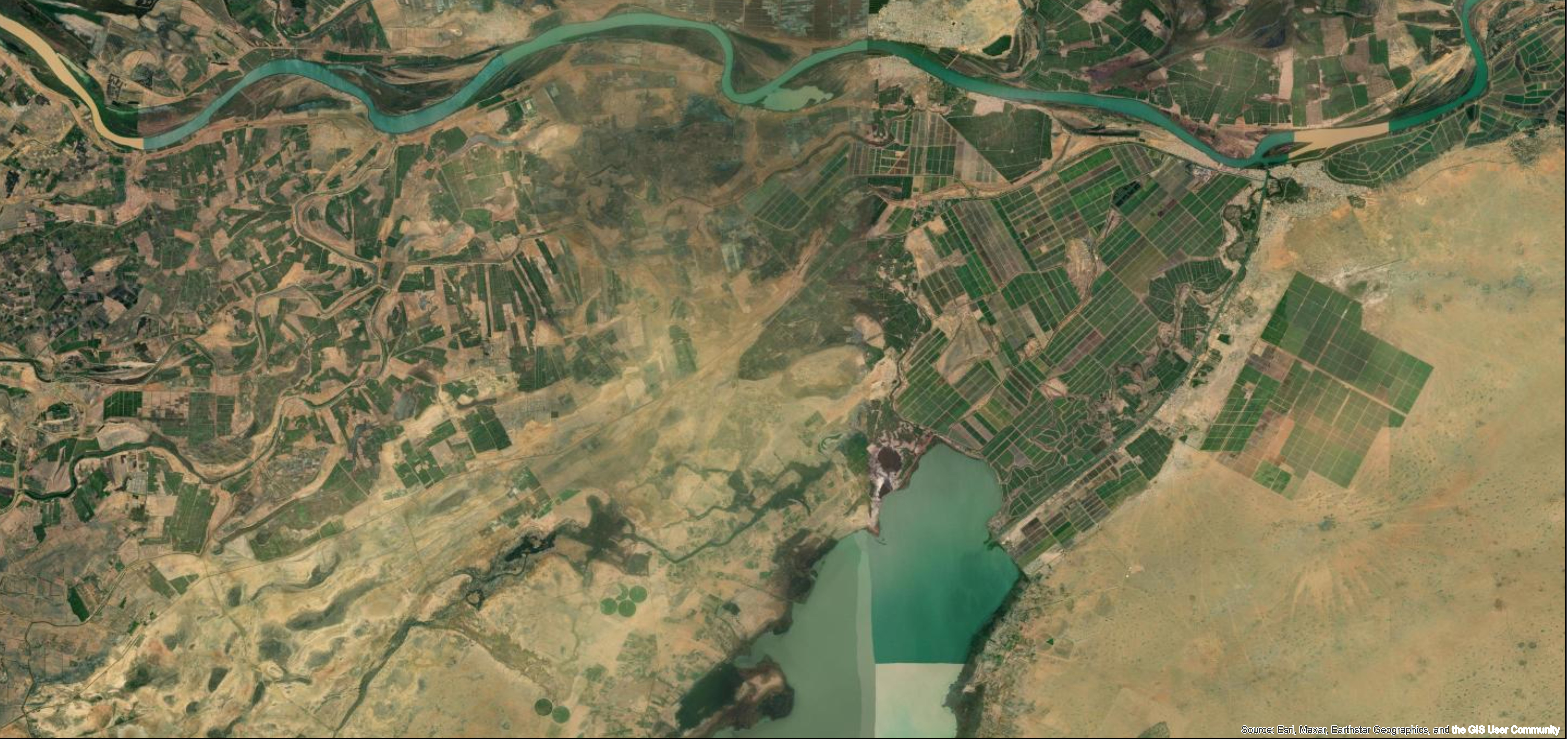
Researchers from the Indian Agricultural Research Institute (ICAR), International Maize and Wheat Improvement Center (CIMMYT) and International Crops Research Institute for the Semi-Arid Tropics (ICRISAT) undertook a study that outlines the different machine learning methods available to explain on-farm yield variability and to demonstrate the practicality of these interpretable techniques for agronomic studies. The study used extensive data for irrigated wheat in the north-western Indo-Gangetic Plains (IGP) of India.
Research revealed the most important management variables explaining yield variability were nitrogen application rate and crop residue management. The average monthly cumulative solar radiation during February and March, during the reproductive phase of the crop, was found to be the most crucial biophysical variable. The data also highlighted the correlation between crop residue management and disease management and nitrogen application rates and seeding rates.
"The study also compared machine learning model outputs against empirical data to contextualize results and provide a blueprint for future analysis in other production systems, " said Dr ML Jat, Global Research Program Director, Resilient Farm and Food Systems, ICRISAT.
To read more, click here